“Speech neuroprosthesis” moves one step closer to becoming a viable, natural communication device for people who have lost the ability to speak.
In an important milestone for the field of brain-computer interfaces (BCIs), researchers at UC San Francisco and UC Berkeley have shown that a speech-controlled BCI can be used to spell out intended sentences from a large vocabulary in real time with 94% accuracy.
The findings, published Nov 8 in Nature Communications, expand on previous work from a clinical trial led by UCSF neurosurgeon Edward Chang, MD, which demonstrated it was possible to decode full words and sentences directly from neural signals sent from the brain to the vocal tract.
In the previous work, a high-density electrocorticography (ECoG) array was implanted over the sensorimotor cortex of a man who had suffered a severe brainstem stroke and subsequently lost his ability to produce intelligible speech. In that study, Chang and his team developed a computer algorithm to decode neural signals corresponding to a vocabulary of 50 words, which could translate the signals into text on a screen as the man attempted to say the words out loud.
In the new study, the same man attempted to silently spell words using the NATO phonetic alphabet (Alfa for A, Beta for B, etc.). The researchers chose the NATO alphabet after discovering the signal was stronger and led to greater accuracy than attempting to spell letters alone. When he attempted to squeeze his hand, the system could also detect that attempt at movement from his brain activity and translate that signal as the end of the sentence.
“The biggest advance over our previous work is the increase in vocabulary size with this new spelling approach,” says David Moses, PhD, postdoctoral engineer in the Chang lab and co-leader of the study. “Although he’s now spelling out the sentences letter-by-letter, our participant has access to over 1,000 words, and in offline analyses we showed that the system can generalize to over 9,000 words, which exceeds the threshold for basic fluency in English.”
This threshold is highly meaningful because it shows that the technology is at least on par with other assistive-communication devices such as eye-tracking devices, which are already used by patients who have lost the ability to speak or type.
“While the initial 50-word vocabulary in our proof-of-concept study was effective with our participant, the spelling BCI allows him to fully express himself in his own words and at a much faster rate than the keyboard device he uses in his day-to-day life,” says Sean Metzger, another co-leader of the study and a graduate student in the UCSF-UC Berkeley Joint Ph.D. Program in Bioengineering.
As speech is a more innate form of communication than writing or typing, the hope is that further development of this technology will enable more rapid and natural expression. The researchers posit that a system combining spelling with direct decoding of whole words will allow for greater flexibility and utility in day-to-day use.
The current study also proves that the system can decode silent attempts at speech without the need for vocal output. “The effort to try to vocalize can be very fatiguing for people with speech paralysis, so silently mouthing the words helps them to flow faster and is less taxing,” says Metzger. “It may also allow us to expand the technology to a wider pool of users and offer hope for individuals who are not able to produce any sounds at all.”
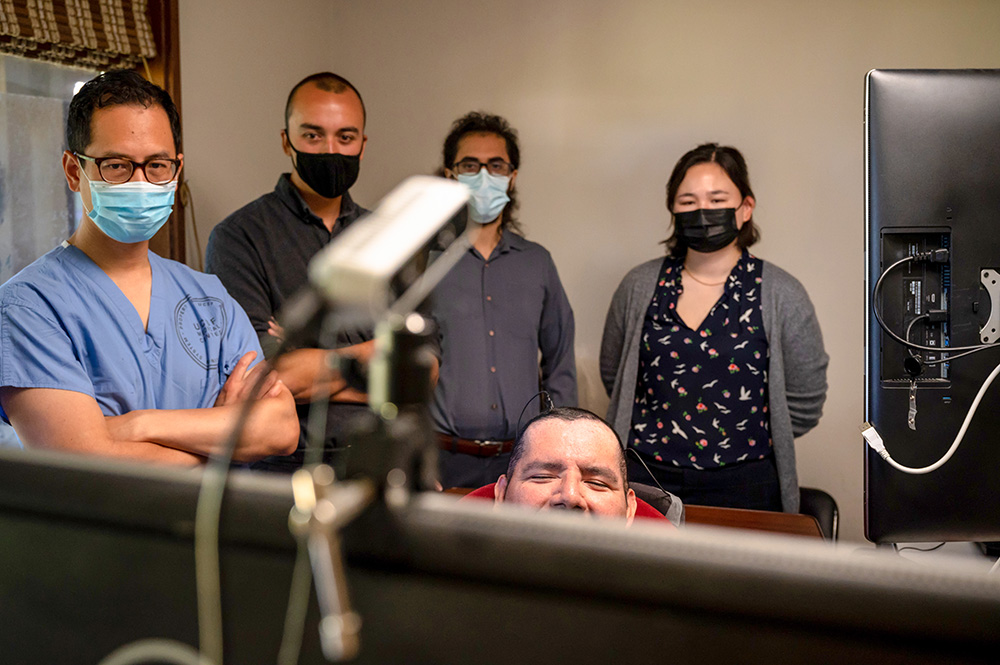
Leveraging Low Frequency
Decoding speech from neuronal activity typically relies on capturing the “high-gamma” features of that activity – information found in the high-gamma frequency range (between 70 and 170 Hz). However, in this new study, the investigators also included an alternative feature set that included low-frequency signals (between 0 and 17 Hz) in order to capture more information from the ECoG array.
“We were surprised by how much that helped our decoders,” says Moses. “The performance nearly doubled when using both features compared to only using the traditional “high-gamma” features. As we continue to refine how we process brain signals to enable the best decoders, we hope that this will inform our future work and the work of other researchers in the field.”
Reference
Sean L. Metzger,* Jessie R. Liu,* David A. Moses,* Maximilian E. Dougherty, Margaret P. Seaton , Kaylo T. Littlejohn, Josh Chartier, Gopala K. Anumanchipalli, Adelyn Tu-Chan, Karunesh Ganguly, & Edward F. Chang. Generalizable spelling using a speech neuroprosthesis in an individual with severe limb and vocal paralysis. Nature Communications. Published online Nov 8 2022. DOI: 10.1038/s41467-022-33611-3.
*These authors contributed equally